Agent2Agent and MCP: An End-to-End Tutorial for a complete Agentic Pipeline
A step-by-step guide to building a complete agentic pipeline using Agent2Agent and MCP
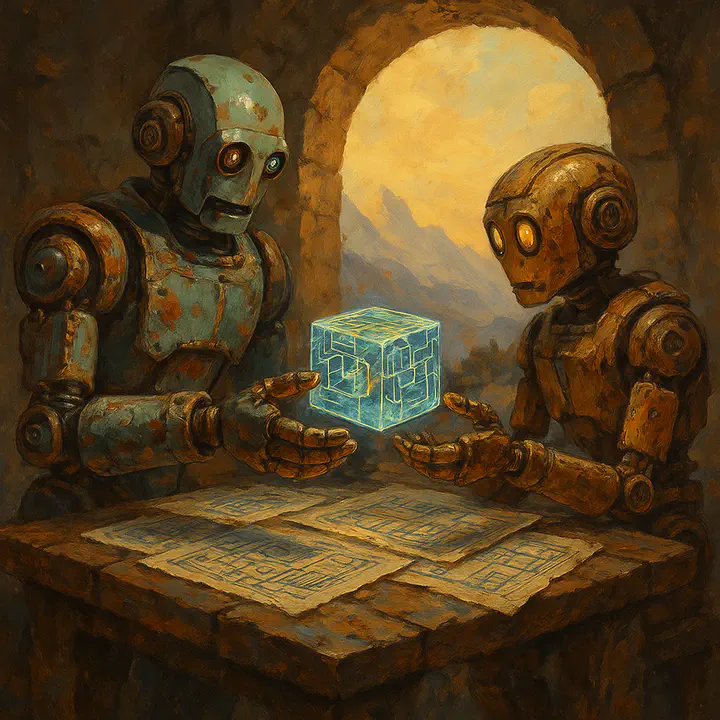
Introduction
In the fast-moving world of agentic AI, two open protocols quietly solve the headaches that used to keep multi-agent projects from ever leaving the lab.
A few months ago, Anthropic introduced Model Context Protocol (MCP): A reliable access to the data and tools an agent needs once the conversation begins. Anthropic describes MCP as a USB-C port for language models—a single, well-defined connector that lets you plug the same model into GitHub, a Postgres database, or a custom knowledge base without rewriting the integration each time. By standardizing the way hosts, clients, and servers exchange “Tools,” “resources,” and “prompts,” MCP turns context into something the model can count on rather than something developers keep stitching together ad hoc.  
Agent2Agent (A2A), a new protocol by Google, tackles another obstacle: getting autonomous agents—often built on different frameworks and hosted in different places—to understand one another. Instead of brittle, one-off bridges, A2A gives every agent a tiny “agent card” that advertises its skills and an HTTP interface that lets other agents negotiate tasks, stream intermediate results, and hand over artifacts. Google started the project to give agents a common language regardless of vendor, and the open-source spec already shows how discovery, task life-cycle updates, and secure push notifications can work out of the box. 
With A2A handling how agents talk to one another and MCP handling how they tap into the outside world, you end up with small, focused agents who can coordinate fluently and still see the bigger picture—an architecture that feels less like a collection of scripts and more like a cooperative workforce.
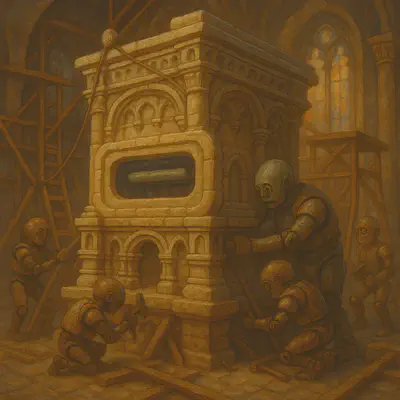
The Structure of this Tutorial
This tutorial will build a complete agentic pipeline using Agent2Agent and MCP. First, we will create and test a couple of simple MCP servers. Then, we will create a simple agent that uses MCP to get information. Finally, we will have a whole crew of agents that uses Agent2Agent to coordinate with each other while using MCP to get information.
For the sake of simplicity, we will use elementary agents that have access to single MCPs and MCPs that perform easy operations like fetching data from an API or searching the web.
We imagine we want to create a team of agents able to make simple reports about American Companies; in particular, we want to be able to ask questions such as “What is the price of the stocks of the top 10 companies in the S&P 500?” or “What are the top 5 producers of lumber in the US”?
Note on the Code
The code for this tutorial is available on GitHub. You should clone the repository, run the code locally, play around with it, and even modify it. This tutorial has been created by starting from two sources:
While the MCP documentation was terrific, the Google Agent2Agent repository had multiple issues and was not working as expected. As a consequence, I heavily modified the code provided.
How to run it
uv by Astral was used as a Python package and project manager. You can clone the repo, run uv sync
, and then run whatever you need.
MCP Servers
Given our objective, we first define two services:
- A crawler able to search on Google and read web pages
- A stock retriever, able to get the price of a stock at a particular time and other helpful info
The Stock Retriever Service
We define a simple stock retriever service using FinHub APIs. The retriever will return a stock’s current price, min, max opening, and closing price (IMPORTANT: if you use the free version of the APIs, only the US market can be queried) with one endpoint and the stock symbol with the other.
class FinHubService:
def __init__(self):
"""
Initializes the finhubservice.
"""
self.client = finnhub.Client(api_key=os.getenv("FINNHUB_API_KEY"))
def get_symbol_from_query(self, query: str) -> Dict[str,Any]:
"""
Given a query (e.g. name of a company) returns a dictionary with info. Use only if you have no idea about the symbol.
:param query: name of company
:return: dictionary with the response to the query
"""
return self.client.symbol_lookup(
query=query,
)
def get_price_of_stock(self, symbol: str) -> Dict[str,Any]:
"""
Given the symbol of a certain strock, returns the live info about it.
:param symbol: The symbol of a stock, e.g. AAPL
:return: a dictionary containing the current_price, change, %change, day high, low, opening and previous closing price
"""
resp = self.client.quote(symbol)
return {
'current_price': resp['c'],
'change': resp['d'],
'percentage_change': resp['dp'],
'day_high': resp['h'],
'day_low': resp['l'],
'day_open_price': resp['o'],
'previous_close_price': resp['pc'],
}
If we run our code with
service = FinHubService()
print(service.get_price_of_stock("AAPL"))
We get something like:
{'current_price': 199.74, 'change': 6.58, 'percentage_change': 3.4065, 'day_high': 201.59, 'day_low': 195.97, 'day_open_price': 196.12, 'previous_close_price': 193.16}
The Crawler Service
Now that we have our Retriever, we want a very simple crawler to search on Google for information and read the web pages returned as results.
class SerperDevService:
def __init__(self):
self.__api_key__=os.getenv("SERPER_DEV_API_KEY")
self.search_url = "https://google.serper.dev/search"
self.scraper_url = "https://scrape.serper.dev"
def search_google(
self,
query: str,
n_results: int = 10,
page: int = 1,
) -> List[Dict[str, Any]]:
"""
Search Google using the Serper.dev API.
:param query: the query to search on google
:param n_results: number of results to return per page
:param page: page number to return
:return: a list of dictionaries containing the search results
"""
payload = json.dumps(
{
"q": query,
"num": n_results,
"page": page
},
)
headers = {
'X-API-KEY': self.__api_key__,
'Content-Type': 'application/json'
}
response = requests.request(
method="POST",
url=self.search_url,
headers=headers,
data=payload,
)
return response.json()['organic']
def get_text_from_page(self, url_to_scrape: str) -> str:
"""
Get text from a page using the Serper.dev API.
:param url_to_scrape: the url of the page to scrape
:return: the text content of the page
"""
payload = json.dumps(
{
"url": url_to_scrape,
}
)
headers = {
'X-API-KEY': self.__api_key__,
'Content-Type': 'application/json'
}
response = requests.request(
method="POST",
url=self.scraper_url,
headers=headers,
data=payload,
)
return response.text
From Service to Server
It is now time to transform those two services into MCP server(s). Given the limited capabilities of the agents in this tutorial, we could create a single MCP server that provides all the tools needed by the agent(s). Nonetheless, the goal here is not to have the best production-ready solution, quite the contrary, but on the other hand to experiment: for this reason, we will create two servers, one per service.
Within the Model Context Protocol, servers come in two flavors distinguished by their transport layer. An STDIO MCP server runs as a local subprocess and pipes JSON-RPC messages over its own stdin/stdout streams, giving minimal latency, full-duplex messaging, and zero network dependencies: it is ideal for command-line tools or same-machine integrations. A Server-Sent Events (SSE) MCP server instead exposes an HTTP endpoint: the client sends requests with lightweight POSTs while the server pushes results back on a single SSE stream, making it naturally web-friendly and accessible across networks or from a browser. In practice, stdio is the lean, no-frills option when everything lives on one host, whereas SSE trades a bit of HTTP overhead and one-way streaming semantics for firewall traversal, browser compatibility, and remote reach.
Given our use case, it seems natural to use the first solution (but we will discover it is not that simple).
mcp = FastMCP("Search Engine Server")
search_service = SerperDevService()
@mcp.tool()
def search_google(
query: str,
n_results: int = 10,
page: int = 1,
) -> list:
"""
Search Google using the Serper.dev API.
:param query: the query to search on google
:param n_results: number of results to return per page
:param page: page number to return
:return: a list of dictionaries containing the search results
"""
return search_service.search_google(query, n_results, page)
@mcp.tool()
def get_text_from_page(url_to_scrape: str) -> str:
"""
Get text from a page using the Serper.dev API.
:param url_to_scrape: the url of the page to scrape
:return: the text content of the page
"""
return search_service.get_text_from_page(url_to_scrape)
if __name__ == "__main__":
mcp.run(transport='stdio')
The code is straightforward (and highly similar to FastAPI, not surprisingly, given that it is used to implement this MCP library).
We first initialise the MCP server with mcp = FastMCP("<NAME_OF_SERVER>")
, then, we define the endpoint/tools using the decorator @mcp.tool()
.
We define the Stocks scraper MCP server in the very same way.
Let’s use the MCP Server
So, at this point we have our working MCP server and we just need to use it. Given this tutorial is about A2A Framework as well, and A2A has been created by Google, we will use the new google ADK to create our agents. To start, we create a single, simple agent that use our MCP server to search the web for information.
We first create a function that spawns our MCP server and “transforms” it into a tool for our ADK agent.
async def get_tools_async():
"""Gets tools from the Search MCP Server."""
print("Attempting to connect to MCP Filesystem server...")
tools, exit_stack = await MCPToolset.from_server(
connection_params=StdioServerParameters(
command="/opt/homebrew/bin/uv", # on macos you need to use this path
args=[
"--directory",
"/root/path/to/mcp_server",
"run",
"search_server.py"
],
env={
"PYTHONPATH": <YOUR_PYTHONPATH_IF_NEEDED>
},
)
)
print("MCP Toolset created successfully.")
return tools, exit_stack
This function will spawn our MCP server and return the tools and the exit stack.
Now, we can create our agent in a similar fashion.
async def get_agent_async():
"""Creates an ADK Agent equipped with tools from the MCP Server."""
tools, exit_stack = await get_tools_async()
print(f"Fetched {len(tools)} tools from MCP server.")
root_agent = LlmAgent(
model='gemini-2.5-pro-exp-03-25',
name='search_agent',
description="Agent to answer questions using Google Search.",
instruction="You are an expert researcher. When someone asks you something you always double check online. You always stick to the facts.",
tools=tools, #
)
return root_agent, exit_stack
This function will create an ADK agent equipped with the tools from the MCP server.
We can now put everything together and create our agent pipeline.
async def async_main():
session_service = InMemorySessionService()
artifacts_service = InMemoryArtifactService()
print("Creating session...")
session = session_service.create_session(
state={}, app_name='mcp_search_app', user_id='searcher_usr', session_id='searcher_session'
)
print(f"Session created with ID: {session.id}")
query = "What are the most tipical sports of the Aosta Valley? Answer with a lot of details."
print(f"User Query: '{query}'")
content = types.Content(role='user', parts=[types.Part(text=query)])
root_agent, exit_stack = await get_agent_async()
runner = Runner(
app_name='mcp_search_app',
agent=root_agent,
artifact_service=artifacts_service,
session_service=session_service,
)
print("Running agent...")
events_async = runner.run_async(
session_id=session.id, user_id=session.user_id, new_message=content
)
async for event in events_async:
if event.is_final_response():
if event.content and event.content.parts:
final_response_text = event.content.parts[0].text
elif event.actions and event.actions.escalate: # Handle potential errors/escalations
final_response_text = f"Agent escalated: {event.error_message or 'No specific message.'}"
print(f"############# Final Response #############\n\n{final_response_text}")
break
print("Closing MCP server connection...")
await exit_stack.aclose()
print("Cleanup complete.")
This function will create a session, run the agent, and print the final response (If you are interested in the answer to the question about the Aosta Valley, you can see below).
If you are interested in the answer to the question about the Aosta Valley, click here
Okay, here are the traditional sports specific to the Aosta Valley, with detailed explanations of how they are played:The Aosta Valley boasts several unique traditional sports, deeply rooted in its rural history and culture, primarily played in spring and autumn. The main ones are Tsan, Rebatta, Fiolet, and Palet. Additionally, the game of Morra is also a popular traditional pastime.
Tsan: Often compared vaguely to baseball or Romanian Oina, Tsan (meaning “field” in the local dialect) is a team sport played on a large grass field, ideally at least 135 meters long. Two teams of 12 players compete. The game involves two main phases. In the first phase (‘battià’ or ’tsachà’), players from one team take turns hitting a small ball (’tsan’) placed in a notch (’t aspot’) on a long wooden or plastic pole (‘percha’ or ‘pertse’) which rests obliquely on a stand (‘bes’). The hitter uses a wooden stick (‘baquet’) to strike the end of the ‘percha’, launching the ’tsan’ into the air, and then hits the airborne ’tsan’ with the ‘baquet’ to send it downfield. The opposing team is spread across the field trying to intercept the ’tsan’ before it lands, primarily using wooden paddles (‘pilon’ or ‘boquet’), which they can even throw to block the ball. A hit is considered ‘good’ (‘bon’) if it lands within the designated field boundaries and is not intercepted. The batting player continues until their shot is intercepted, or they hit the ’tsan’ out of bounds three consecutive times or four times in total. The number of ‘bone’ (good hits) each player accumulates is tallied for the team. After all players on the first team have batted, the teams switch roles. In the second phase (‘servià’ or ‘paletà’), each player must convert their accumulated ‘bone’ into distance. A player from the opposing team throws the ’tsan’ high in the air towards the original batting area. The player whose turn it is attempts to hit this thrown ’tsan’ with a different wooden paddle (‘paleta’ or ‘piota’), aiming for maximum distance. The distance of this hit is measured in meters. Finally, the total meters achieved by all players on each team are summed up. The team that accumulates an advantage of at least 40 meters over the opponent wins the match; if the difference is less, it’s a draw (though in finals or play-offs, a single meter advantage is enough to win). Due to the hardness of the wooden ’tsan’, players often wear helmets for safety.
Rebatta: This game belongs to the family of bat-and-ball sports, like golf or baseball. It involves hitting a small ball (‘rebatta’, made of wood studded with nails or metal, about 30mm diameter) as far as possible. To do this, the player uses two specific wooden tools. First is the ‘fioletta’, a pipe-shaped lever about 20cm long. The ‘rebatta’ is placed on the slightly hollowed end of the ‘fioletta’ which rests on the ground. The player then strikes the other end of the ‘fioletta’ with a long wooden club (‘masetta’, 100-140cm long with a distinct head called ‘maciocca’) making the ‘fioletta’ act as a lever to pop the ‘rebatta’ into the air (this action is called ’levoù’). Immediately after, the player swings the ‘masetta’ again to strike the airborne ‘rebatta’ forcefully, sending it downfield. The playing field is typically an isosceles triangle marked out on grass, with the vertex at the batting spot (‘place’). The length varies by category but can be up to 240 meters. Lines are marked across the field every 15 meters, and landing the ‘rebatta’ beyond a line scores a corresponding number of points (1 point for passing the first line, 2 for the second, etc.). Matches are often played between teams (e.g., five players each), competing over a set number of turns (‘bars’), with both team and individual championships held.
Fiolet: Similar in objective to Rebatta (hitting an object for maximum distance), Fiolet uses slightly different equipment and technique. The object hit is the ‘fiolet’ itself, which is ovoid-shaped with one slightly flattened part. Instead of using a separate lever like Rebatta’s ‘fioletta’, the ‘fiolet’ is balanced directly on a smooth stone (‘pira’) or a purpose-made stand. The player uses a bat (also often called ‘masetta’ or ‘baton’) to strike the edge of the balanced ‘fiolet’ skillfully. This initial impact makes the ‘fiolet’ jump vertically into the air. The player then takes a full swing with the bat to hit the airborne ‘fiolet’ and drive it as far as possible down the field, potentially over 150 meters. Like Rebatta, scoring is based on the distance achieved, often measured in zones or points corresponding to set distances. Competitions include team events (often 5 players per team) and a notable individual championship where the winner receives the “Baton d’Or” (Golden Bat) trophy for the year.
Palet: This sport is akin to horseshoes or the French ‘jeu de palets’. Players throw heavy, round, flat metal discs called ‘palets’ towards a smaller target pin, known as the ‘bolin’ or ‘billet’, which is placed at a distance (often around 10-20 meters) on a designated court, usually made of clay or packed earth. The objective is to land your ‘palet’ as close to the ‘bolin’ as possible. Players typically throw a set number of ‘palets’ per round. Scoring occurs after all ‘palets’ are thrown in a round. Points are awarded to the player or team whose ‘palet(s)’ are closest to the ‘bolin’. Usually, only the ‘palets’ closer than the opponent’s closest ‘palet’ can score. It requires precision and strategy in throwing the heavy discs. It’s played both individually and in teams, often in dedicated courts found throughout the valley.
Morra (or Moura): While simpler and often considered more of a game than a sport requiring a dedicated field, Morra is a very traditional and lively pastime in the Aosta Valley. It’s typically played between two people. Both players simultaneously extend one hand, showing a number of fingers from 1 to 5 (or a closed fist representing zero). At the same instant they extend their hand, each player loudly shouts out their guess for the total sum of fingers shown by both players combined (so the guess will be between 0 and 10). The player who correctly guesses the sum scores a point. The game is fast-paced, often played in rounds to a set score, and is known for the animated shouts and gestures involved.
Multiple Local MCP Servers: where STDIO is not enough
Now, we have a single MCP server that can be used by our agent. However, especially if we plan to play with multiple agents, we want to have multiple MCP servers, each one with a specific purpose. For example, we want one MCP server to be able to search the web, another to retrieve stocks, and so on.
If you try to spawn multiple STDIO-based MCP servers, you will notice that they will not work at the same time. To solve this problem, we can use an SSE-based MCP server with some tricks.
It is quite simple: we declare the “endpoints” as before,
@mcp.tool()
def search_google(
query: str,
n_results: int = 10,
page: int = 1,
) -> list:
"""
Search Google using the Serper.dev API.
:param query: the query to search on google
:param n_results: number of results to return per page
:param page: page number to return
:return: a list of dictionaries containing the search results
"""
return search_service.search_google(query, n_results, page)
@mcp.tool()
def get_text_from_page(url_to_scrape: str) -> str:
"""
Get text from a page using the Serper.dev API.
:param url_to_scrape: the url of the page to scrape
:return: the text content of the page
"""
return search_service.get_text_from_page(url_to_scrape)
Then, we wrap the server in a Startlette app:
def create_starlette_app(
mcp_server: Server,
*,
debug: bool = False,
) -> Starlette:
"""
Create a Starlette application that can server the provied mcp server with SSE.
:param mcp_server: the mcp server to serve
:param debug: whether to enable debug mode
:return: a Starlette application
"""
sse = SseServerTransport("/messages/")
async def handle_sse(request: Request) -> None:
async with sse.connect_sse(
request.scope,
request.receive,
request._send,
) as (read_stream, write_stream):
await mcp_server.run(
read_stream,
write_stream,
mcp_server.create_initialization_options(),
)
return Starlette(
debug=debug,
routes=[
Route("/sse", endpoint=handle_sse),
Mount("/messages/", app=sse.handle_post_message),
],
)
We can then run each server with a different port as follows:
mcp_server = mcp._mcp_server # noqa: WPS437
parser = argparse.ArgumentParser(description='Run MCP SSE-based server')
parser.add_argument('--host', default='0.0.0.0', help='Host to bind to')
parser.add_argument('--port', type=int, default=8080, help='Port to listen on')
args = parser.parse_args()
starlette_app = create_starlette_app(mcp_server, debug=True)
uvicorn.run(starlette_app, host=args.host, port=args.port)
To pass such a server to an ADK agent, as a tool we’ll need something like:
async def return_sse_mcp_tools_search():
print("Attempting to connect to MCP server for search and page read...")
server_params = SseServerParams(
url="http://localhost:<CHOSEN_PORT>/sse",
)
tools, exit_stack = await MCPToolset.from_server(connection_params=server_params)
print("MCP Toolset created successfully.")
return tools, exit_stack
Multiple Agents
It is now time to create a more complex pipeline with multiple agents. At first, we will create a hierarchical crew of agents using the ADK library by Google, then, after seeing that our solution works, we will use Agent2Agent framework to create peer-to-peer agents able to coordinate with each other.
Hierarchical Crew
Now that we have our MCP servers set up, we can create a hierarchical crew of agents using the ADK library. In this example, we’ll create a team of agents that can analyze companies and their stocks, with a main coordinator agent delegating tasks to specialized sub-agents.
Setting Up the Crew
First, we define some constants for our application:
MODEL = 'gemini-2.5-pro-exp-03-25'
APP_NAME = 'company_analysis_app'
USER_ID = 'searcher_usr'
SESSION_ID = 'searcher_session'
These constants define the model we’ll use (Gemini 2.5 Pro), the application name, and session identifiers.
Creating Specialized Agents
We create two specialized agents, each with their own set of tools:
- Stock Analysis Agent: This agent is responsible for analyzing stock data and providing insights about stock prices and market performance.
stock_analysis_agent = Agent(
model=MODEL,
name="stock_analysis_agent",
instruction="Analyze stock data and provide insights.",
description="Handles stock analysis and provides insights, in particular, can get the latest stock price.",
tools=stocks_tools,
)
- Search Agent: This agent specializes in searching the web and reading online content.
search_agent = Agent(
model=MODEL,
name="search_agent",
instruction="Expert googler. Can search anything on google and read pages online.",
description="Handles search queries and can read pages online.",
tools=search_tools,
)
The Root Agent
The root agent acts as the coordinator, delegating tasks to the specialized agents based on the user’s query:
root_agent = Agent(
name="company_analysis_assistant",
model=MODEL,
description="Main assistant: Handles requests about stocks and information of companies.",
instruction=(
"You are the main Assistant coordinating a team. Your primary responsibilities are providing company and stocks reports and delegating other tasks.\n"
"1. If the user asks about a company, provide a detailed report.\n"
"2. If you need any information about the current stock price, delegate to the stock_analysis_agent.\n"
"3. If you need to search for information, delegate to the search_agent.\n"
"Analyze the user's query and delegate or handle it appropriately. If unsure, ask for clarification. Only use tools or delegate as described."
),
sub_agents=[search_agent, stock_analysis_agent],
output_key="last_assistant_response",
)
The root agent’s instruction clearly defines its role and how it should delegate tasks to its sub-agents. It’s designed to:
- Provide detailed company reports
- Delegate stock price queries to the stock analysis agent
- Delegate search queries to the search agent
- Ask for clarification when needed
Running the Crew
The main function sets up the session and runs the agent pipeline:
async def async_main():
# Initialize services
session_service = InMemorySessionService()
artifacts_service = InMemoryArtifactService()
# Create session
session = session_service.create_session(
state={},
app_name=APP_NAME,
user_id=USER_ID,
session_id=SESSION_ID,
)
# Get user query
query = input("Enter your query:\n")
content = types.Content(role='user', parts=[types.Part(text=query)])
# Initialize tools from MCP servers
search_tools, search_exit_stack = await return_sse_mcp_tools_search()
stocks_tools, stocks_exit_stack = await return_sse_mcp_tools_stocks()
# Create and run the agent pipeline
runner = Runner(
app_name=APP_NAME,
agent=root_agent,
artifact_service=artifacts_service,
session_service=session_service,
)
# Process events and get final response
events_async = runner.run_async(
session_id=session.id,
user_id=session.user_id,
new_message=content
)
async for event in events_async:
if event.is_final_response():
if event.content and event.content.parts:
final_response_text = event.content.parts[0].text
elif event.actions and event.actions.escalate:
final_response_text = f"Agent escalated: {event.error_message or 'No specific message.'}"
print(colored(text=f"############# Final Response #############\n\n{final_response_text}", color='green'))
break
else:
print(event)
# Cleanup
await stocks_exit_stack.aclose()
await search_exit_stack.aclose()
This implementation creates a hierarchical crew where:
- The root agent receives the user’s query
- It analyzes the query and decides whether to:
- Handle it directly
- Delegate to the stock analysis agent
- Delegate to the search agent
- The specialized agents use their respective MCP tools to gather information
- The root agent coordinates the responses and provides a final answer to the user
The crew will automatically delegate tasks to the appropriate agents and provide comprehensive responses combining information from both the stock market and web searches.
Agent2Agent Crew
Now, it’s time to finally play with the Agent2Agent framework. As we previously said, A2A is a framework that allows agents to coordinate with each other in a peer-to-peer manner by using a standardised interface.
The said agents can be implemented in any language or framework that supports the A2A interface. In this example, we will use the ADK library by Google to create the agents for simplicity.
A further disclaimer: at the time of writing, the A2A implementations i’ve found online, comprised the one from google, are quite raw, for this example I have heavily takend and modified the code from the A2A tutorial from the official repository.
The Main Conceptual Components
The Agent2Agent (A2A) protocol enables seamless interaction between autonomous AI agents. Here are its fundamental building blocks:
Agent Card: A standardized metadata document (typically located at /.well-known/agent.json
) that serves as an agent’s digital identity card. It details the agent’s capabilities, available skills, service endpoint, and security requirements, enabling other agents to discover and understand how to interact with it.
A2A Server: An agent that implements the A2A protocol by exposing an HTTP endpoint. It handles incoming requests, manages task execution, and maintains communication with clients according to the protocol specifications.
A2A Client: Any application or agent that consumes A2A services. It initiates interactions by sending requests (such as tasks/send
or tasks/sendSubscribe
) to an A2A Server’s endpoint.
Task: The primary unit of work in A2A. When a client initiates a task, it creates a unique conversation thread that can progress through various states: submitted, working, input-required, completed, failed, or canceled. Each task maintains its own context and history.
Message: The basic unit of communication between clients and agents. Messages contain a role (either “user” or “agent”) and are composed of one or more Parts, forming the conversation thread.
Part: The atomic content unit within Messages or Artifacts. Parts can be of different types:
- TextPart: For plain text content
- FilePart: For file data (either inline or referenced via URI)
- DataPart: For structured JSON data (commonly used for forms or structured responses)
Artifact: Represents any output generated by an agent during task execution. This could include generated files, structured data, or other resources. Like Messages, Artifacts are composed of Parts.
Streaming: For tasks that require extended processing time, servers can implement streaming capabilities through tasks/sendSubscribe
. This allows clients to receive real-time updates via Server-Sent Events (SSE), including task status changes and new artifacts.
Push Notifications: Servers supporting this feature can proactively notify clients about task updates through a webhook URL. Clients configure their notification endpoint using tasks/pushNotification/set
.
Typical Interaction Flow
Discovery: The client retrieves the Agent Card from the server’s well-known URL to understand the agent’s capabilities and requirements.
Initiation: The client starts a new task by sending a
tasks/send
ortasks/sendSubscribe
request, including the initial message and a unique Task ID.Processing:
- In streaming mode: The server sends real-time updates via SSE, including status changes and new artifacts
- In non-streaming mode: The server processes the task synchronously and returns the final result
Interaction: If the task requires additional input, the client can send follow-up messages using the same Task ID.
Completion: The task eventually reaches a final state (completed, failed, or canceled), concluding the interaction.
The ADKAgent Class
Now that we understand the conceptual components of A2A, let’s look at how we can implement it using the ADK library. The following code shows how to create an agent that can both act as a standalone agent and coordinate with other agents in the network.
The ADKAgent
class serves as the foundation for our A2A implementation. It bridges the Google ADK framework with the A2A protocol, allowing agents to communicate and coordinate tasks. Here’s a breakdown of its key components:
class ADKAgent:
"""An agent that handles stock report requests."""
SUPPORTED_CONTENT_TYPES = ["text", "text/plain"]
def __init__(
self,
model: str,
name: str,
description: str,
instructions: str,
tools: List[Any],
is_host_agent: bool = False,
remote_agent_addresses: List[str] = None,
task_callback: TaskUpdateCallback | None = None
):
# ... initialization code ...
The class can be configured in two modes:
- Standalone Agent: When
is_host_agent=False
, it acts as a regular ADK agent with its own tools and capabilities - Host Agent: When
is_host_agent=True
, it becomes a coordinator that can delegate tasks to other agents
Key Features
- Remote Agent Management:
def register_agent_card(self, card: AgentCard):
remote_connection = RemoteAgentConnections(card)
self.remote_agent_connections[card.name] = remote_connection
self.cards[card.name] = card
This method allows the agent to discover and connect to other agents in the network. Each agent’s capabilities are described in its AgentCard
, which is stored in the .well-known/agent.json
file.
- Task Delegation:
async def send_task(
self,
agent_name: str,
message: str,
tool_context: ToolContext
):
# ... task delegation code ...
The send_task
method handles the core A2A protocol interaction. It:
- Creates a new task with a unique ID
- Sends the task to the specified remote agent
- Manages the task lifecycle (submitted, working, completed, etc.)
- Handles responses and artifacts from the remote agent
- State Management:
def check_state(self, context: ReadonlyContext):
state = context.state
if ('session_id' in state and
'session_active' in state and
state['session_active'] and
'agent' in state):
return {"active_agent": f'{state["agent"]}'}
return {"active_agent": "None"}
The agent maintains state information about:
- Active sessions
- Current tasks
- Connected agents
- Conversation context
- Response Processing:
def convert_parts(parts: list[Part], tool_context: ToolContext)
def convert_part(part: Part, tool_context: ToolContext)
These methods handle the conversion between different response formats, supporting:
- Text responses
- Structured data
- File attachments
- Artifacts
Usage Example
Here’s how you can use the ADKAgent
to create a coordinator agent that delegates tasks to specialized agents:
# Create a host agent
host_agent = ADKAgent(
model="gemini-2.5-pro",
name="coordinator",
description="Main coordinator agent",
instructions="Coordinate tasks between agents",
tools=[],
is_host_agent=True,
remote_agent_addresses=["http://agent1:8080", "http://agent2:8080"]
)
The Agent Card Class
In the previous piece of code, we have seen a AgentCard
object being used in many places.
The AgentCard
class is a crucial component of the Agent2Agent (A2A) protocol, serving as a standardized way to describe an agent’s capabilities and requirements. It’s essentially an agent’s digital identity card that other agents can use to discover and understand how to interact with it.
Let’s break down the key components of the generate_agent_card
function:
Basic Agent Information:
agent_name
: A unique identifier for the agentagent_description
: A human-readable description of what the agent doesagent_url
: The endpoint where the agent can be reachedagent_version
: The version of the agent implementation
Capabilities:
can_stream
: Indicates if the agent supports streaming responses via Server-Sent Events (SSE)can_push_notifications
: Specifies if the agent can send push notificationscan_state_transition_history
: Determines if the agent maintains a history of state transitions
Communication Settings:
authentication
: Defines the authentication method required to interact with the agentdefault_input_modes
: Lists the supported input formats (e.g., “text”, “json”)default_output_modes
: Lists the supported output formats
Skills:
skills
: A list ofAgentSkill
objects that describe the specific capabilities of the agent
Here’s an example of how to use the generate_agent_card
function to create a card for a stock analysis agent:
stock_agent_card = generate_agent_card(
agent_name="stock_analyzer",
agent_description="Analyzes stock market data and provides insights",
agent_url="http://localhost:8080",
agent_version="1.0.0",
can_stream=True,
can_push_notifications=True,
skills=[
AgentSkill(
name="stock_analysis",
description="Analyzes stock prices and market trends",
input_schema={
"type": "object",
"properties": {
"symbol": {"type": "string"},
"timeframe": {"type": "string"}
}
},
output_schema={
"type": "object",
"properties": {
"price": {"type": "number"},
"trend": {"type": "string"}
}
}
)
]
)
The generated agent card is typically served at the /.well-known/agent.json
endpoint of the agent’s URL. This standardized location allows other agents to discover and understand how to interact with the agent without prior knowledge of its implementation details.
When another agent wants to interact with this agent, it can:
- Fetch the agent card from
/.well-known/agent.json
- Verify that the agent supports the required capabilities
- Check if the agent has the necessary skills
- Use the provided URL and authentication method to establish communication
The A2A Server
To make the agent “contactable”, we need to implement the A2A server. The server is responsible for handling incoming requests from other agents and managing the communication protocol. Here’s a breakdown of the key components:
- Server Initialization:
class A2AServer:
def __init__(
self,
host="0.0.0.0",
port=5000,
endpoint="/",
agent_card: AgentCard = None,
task_manager: TaskManager = None,
):
self.host = host
self.port = port
self.endpoint = endpoint
self.task_manager = task_manager
self.agent_card = agent_card
self.app = Starlette()
self.app.add_route(self.endpoint, self._process_request, methods=["POST"])
self.app.add_route(
"/.well-known/agent.json", self._get_agent_card, methods=["GET"]
)
The server is initialized with:
- Host and port configuration
- An endpoint for handling requests
- An agent card describing the agent’s capabilities
- A task manager for handling task execution
- Request Processing:
async def _process_request(self, request: Request):
try:
body = await request.json()
json_rpc_request = A2ARequest.validate_python(body)
if isinstance(json_rpc_request, GetTaskRequest):
result = await self.task_manager.on_get_task(json_rpc_request)
elif isinstance(json_rpc_request, SendTaskRequest):
result = await self.task_manager.on_send_task(json_rpc_request)
# ... handle other request types ...
The server processes different types of requests:
- Task creation and management
- Streaming task updates
- Push notifications
- Task cancellation
- Asynchronous Server Startup:
async def astart(self):
if self.agent_card is None:
raise ValueError("agent_card is not defined")
if self.task_manager is None:
raise ValueError("request_handler is not defined")
config = uvicorn.Config(self.app, host=self.host, port=self.port, loop="asyncio")
server = uvicorn.Server(config)
# start in the background
server_task = asyncio.create_task(server.serve())
# wait for startup
while not server.started:
await asyncio.sleep(0.1)
print("Server is up – press Ctrl+C to shut it down manually")
try:
await server_task
except KeyboardInterrupt:
server.should_exit = True
await server_task
The astart
method is a crucial modification from the original Google implementation. Here’s why:
- Original Implementation:
def start(self):
if self.agent_card is None:
raise ValueError("agent_card is not defined")
if self.task_manager is None:
raise ValueError("request_handler is not defined")
uvicorn.run(self.app, host=self.host, port=self.port)
The original implementation used a synchronous start
method that blocked the main thread. This was problematic because:
- It couldn’t be integrated into an existing async event loop
- It made it difficult to coordinate with other async components (like MCP tools)
- New Implementation:
The new
astart
method:
- Creates a Uvicorn server with an async event loop
- Starts the server in the background using
asyncio.create_task
- Waits for the server to be fully started
- Handles graceful shutdown on keyboard interrupt
- Can be integrated into an existing async application
This change was necessary because:
- MCP tools are inherently asynchronous
- The server needs to be able to run alongside other async components
- We need proper control over the server lifecycle
- Response Handling:
def _create_response(self, result: Any) -> JSONResponse | EventSourceResponse:
if isinstance(result, AsyncIterable):
async def event_generator(result) -> AsyncIterable[dict[str, str]]:
async for item in result:
yield {"data": item.model_dump_json(exclude_none=True)}
return EventSourceResponse(event_generator(result))
elif isinstance(result, JSONRPCResponse):
return JSONResponse(result.model_dump(exclude_none=True))
The server supports two types of responses:
- Regular JSON responses for immediate results
- Server-Sent Events (SSE) for streaming updates
This implementation allows the A2A server to:
- Handle multiple concurrent requests
- Support streaming responses
- Integrate with async components
- Provide proper error handling
- Support graceful shutdown
The server can now be used in an async context like this:
async def main():
server = A2AServer(
host="0.0.0.0",
port=5000,
agent_card=my_agent_card,
task_manager=my_task_manager
)
await server.astart()
This makes it possible to integrate the A2A server with other async components, such as MCP tools or other agents, while maintaining proper control over the server lifecycle.
The A2A Card Resolver
The A2A Card Resolver is a class that is used to resolve the agent card of an agent. It is used to find the agent card of an agent when it is not known. The code is quite simple:
class A2ACardResolver:
def __init__(self, base_url, agent_card_path="/.well-known/agent.json"):
self.base_url = base_url
self.agent_card_path = agent_card_path.lstrip("/")
def get_agent_card(self) -> AgentCard:
with httpx.Client() as client:
url = re.match(r'(https?://[^/]+)', self.base_url).group(1).rstrip("/")
response = client.get(url + "/" + self.agent_card_path)
response.raise_for_status()
try:
resp_dict = response.json()
resp_dict['url'] = self.base_url
return AgentCard(**resp_dict)
except json.JSONDecodeError as e:
raise A2AClientJSONError(str(e)) from e
For some reason, the original example from Google was not working. since it “destroyed” the url while attaching the card path.
Common Resources, utils and the rest
On top of those main components, in this tutorial we have used a list of other resources, many of them unchanged from the original tutorial: the vast majority can be found here.
Putting everything together
So, now we have all the components we need to put everything together.
We create the Host Agent:
async def run_agent():
AGENT_NAME = "host_agent"
AGENT_DESCRIPTION = "An agent orchestrates the decomposition of the user request into tasks that can be performed by the child agents."
PORT = 12000
HOST = "0.0.0.0"
AGENT_URL = f"http://{HOST}:{PORT}"
AGENT_VERSION = "1.0.0"
MODEL = 'gemini-2.5-pro-preview-03-25'
AGENT_SKILLS = [
AgentSkill(
id="COORDINATE_AGENT_TASKS",
name="coordinate_tasks",
description="coordinate tasks between agents.",
),
]
list_urls = [
"http://localhost:11000/google_search_agent",
"http://localhost:10000/stock_agent",
]
AGENT_CARD = generate_agent_card(
agent_name=AGENT_NAME,
agent_description=AGENT_DESCRIPTION,
agent_url=AGENT_URL,
agent_version=AGENT_VERSION,
can_stream=False,
can_push_notifications=False,
can_state_transition_history=True,
default_input_modes=["text"],
default_output_modes=["text"],
skills=AGENT_SKILLS,
)
host_agent = ADKAgent(
model=MODEL,
name="host_agent",
description="",
tools=[],
instructions="",
is_host_agent=True,
remote_agent_addresses=list_urls,
)
task_manager = generate_agent_task_manager(
agent=host_agent,
)
server = A2AServer(
host=HOST,
port=PORT,
endpoint="/host_agent",
agent_card=AGENT_CARD,
task_manager=task_manager
)
print(f"Starting {AGENT_NAME} A2A Server on {AGENT_URL}")
await server.astart()
The Search Agent:
import asyncio
from typing import List, Any
from dotenv import load_dotenv, find_dotenv
from google.adk import Agent
from google.adk.tools import google_search
from a2a_servers.agent_servers.utils import generate_agent_task_manager, generate_agent_card
from a2a_servers.agents.adk_agent import ADKAgent
from a2a_servers.common.agent_task_manager import AgentTaskManager
from a2a_servers.common.server.server import A2AServer
from a2a_servers.common.types import (
AgentCard,
AgentCapabilities,
AgentSkill,
)
from adk_agents_testing.mcp_tools.mcp_tool_search import return_sse_mcp_tools_search
load_dotenv(find_dotenv())
async def run_agent():
AGENT_NAME = "google_search_agent"
AGENT_DESCRIPTION = "An agent that handles search queries and can read pages online."
HOST = "0.0.0.0"
PORT = 11000
AGENT_URL = f"http://{HOST}:{PORT}"
AGENT_VERSION = "1.0.0"
MODEL = 'gemini-2.5-pro-preview-03-25'
AGENT_SKILLS = [
AgentSkill(
id="GOOGLE_SEARCH",
name="google_search",
description="Handles search queries and can read pages online.",
),
]
AGENT_CARD = generate_agent_card(
agent_name=AGENT_NAME,
agent_description=AGENT_DESCRIPTION,
agent_url=AGENT_URL,
agent_version=AGENT_VERSION,
can_stream=False,
can_push_notifications=False,
can_state_transition_history=True,
default_input_modes=["text"],
default_output_modes=["text"],
skills=AGENT_SKILLS,
)
gsearch_tools, g_search_exit_stack = await return_sse_mcp_tools_search()
google_search_agent = ADKAgent(
model=MODEL,
name="google_search_agent",
description="Handles search queries and can read pages online.",
tools=gsearch_tools,
instructions=(
"You are an expert googler. Can search anything on google and read pages online."
),
)
task_manager = generate_agent_task_manager(
agent=google_search_agent,
)
server = A2AServer(
host=HOST,
port=PORT,
endpoint="/google_search_agent",
agent_card=AGENT_CARD,
task_manager=task_manager
)
print(f"Starting {AGENT_NAME} A2A Server on {AGENT_URL}")
await server.astart()
if __name__ == "__main__":
asyncio.run(
run_agent()
)
And the Stock Agent:
async def run_agent():
AGENT_NAME = "stock_report_agent"
AGENT_DESCRIPTION = "An agent that provides US stock prices and info."
PORT = 10000
HOST = "0.0.0.0"
AGENT_URL = f"http://{HOST}:{PORT}"
AGENT_VERSION = "1.0.0"
MODEL = 'gemini-2.5-pro-preview-03-25'
AGENT_SKILLS = [
AgentSkill(
id="SKILL_STOCK_REPORT",
name="stock_report",
description="Provides stock prices and info.",
),
]
AGENT_CARD = generate_agent_card(
agent_name=AGENT_NAME,
agent_description=AGENT_DESCRIPTION,
agent_url=AGENT_URL,
agent_version=AGENT_VERSION,
can_stream=False,
can_push_notifications=False,
can_state_transition_history=True,
default_input_modes=["text"],
default_output_modes=["text"],
skills=AGENT_SKILLS,
)
stocks_tools, stocks_exit_stack = await return_sse_mcp_tools_stocks()
stock_analysis_agent = ADKAgent(
model=MODEL,
name="stock_analysis_agent",
description="Handles stock analysis and provides insights, in particular, can get the latest stock price.",
tools=stocks_tools,
instructions=(
"Analyze stock data and provide insights. You can also get the latest stock price."
"If the user asks about a company, the stock prices for the said company."
"If the user asks about a stock, provide the latest stock price and any other relevant information."
"You can get only the latest stock price for US companies."
),
)
task_manager = generate_agent_task_manager(
agent=stock_analysis_agent,
)
server = A2AServer(
host=HOST,
port=PORT,
endpoint="/stock_agent",
agent_card=AGENT_CARD,
task_manager=task_manager
)
print(f"Starting {AGENT_NAME} A2A Server on {AGENT_URL}")
await server.astart()
Then, run everything.
First the MCP Servers:
uv run mcp server/sse/search_server.py
uv run mcp server/sse/stocks_server.py
And then the A2A Servers:
uv run a2a_servers/agent_servers/stock_report_agent_server.py
uv run a2a_servers/agent_servers/google_search_agent_server.py
And finally, the Host Agent:
uv run a2a_servers/host_agent_server.py
We can then contact the host agent (e.g, using the script at a2a_servers/run_from_local_client.py
).
Here you can see a video of the whole process: